By Dr. Dwi Suryanto, Management Strategist and Author of Transformational Leadership
I. Introduction: The Day the Nasdaq Trembled
The Nasdaq’s 3% nosedive this week—erasing $600 billion in tech valuations—wasn’t driven by interest rates or inflation, but by a seismic shock from an unassuming source: DeepSeek, a Chinese AI startup that leapfrogged ChatGPT to become Apple’s #1 app. This moment mirrors the 1957 launch of Sputnik, which jolted the U.S. into the space race. For leaders, DeepSeek’s rise is a wake-up call: efficiency-driven disruption is rewriting the rules of global tech competition.
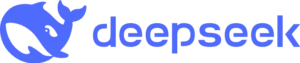
As a management strategist, I see this as a pivotal case study in strategic agility and resource reallocation. Let’s decode what it means for leaders navigating this new frontier.
II. The Efficiency Revolution: When Less Becomes More
Toyota vs. Ford: A Lesson from Automotive History
In the 1980s, Toyota’s lean manufacturing disrupted Detroit’s reliance on scale, proving that doing more with less could outpace brute-force production. DeepSeek’s 5.6 million AI model—developed with basic Nvidia chips—echoes this playbook. Compare this to Meta’s $65 billion AI bet or OpenAI’s opaque billion-dollar budgets, and the contrast is stark.
Open Source as the Great Equalizer
DeepSeek’s MIT-licensed model follows the trajectory of Linux, which democratized software by undermining Microsoft’s closed ecosystems. Today, enterprises like Meta are already pivoting to open-source models (e.g., LLaMA) to reduce dependency on costly proprietary systems. The lesson? Control shifts to those who collaborate, not just those who spend.
Example: Just as Netflix’s streaming model killed Blockbuster’s brick-and-mortar dominance, DeepSeek’s efficiency threatens incumbents wedded to legacy R&D frameworks.
III. Geopolitical Shockwaves: The New Cold War of Code
The Sputnik Parallel
Marc Andreessen’s comparison of DeepSeek to Sputnik is apt—but with a twist. While Sputnik spurred U.S. investment in NASA, DeepSeek’s open-source model globalizes AI innovation, bypassing traditional hardware gatekeepers like ASML or Nvidia. China’s constraints (U.S. chip bans) forced a software-first strategy, mirroring Japan’s 1970s pivot to fuel-efficient cars post-oil crisis.
TikTok vs. DeepSeek: A Tale of Two Threats
While TikTok faces U.S. bans over data privacy, DeepSeek’s open-source code poses a subtler risk: dependency on foreign innovation. Imagine a world where Chinese AI standards dominate, much like Huawei’s 5G infrastructure—this is the geopolitical chessboard now in play.
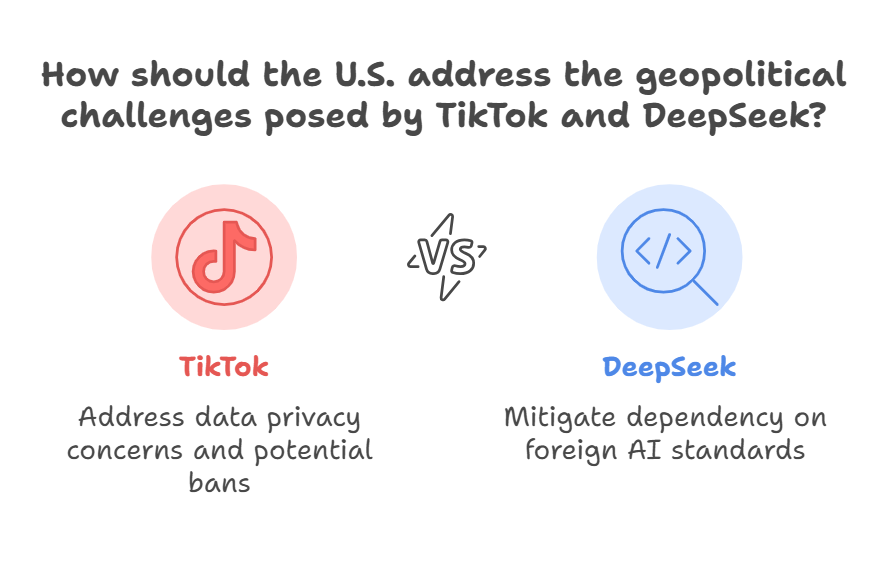
IV. Leadership in Crisis: Microsoft’s $80 Billion Dilemma
The Jevons Paradox: Efficiency’s Double-Edged Sword
Satya Nadella’s tweet invoking the Jevons Paradox—efficiency drives demand, not reduces it—hints at Microsoft’s balancing act. While DeepSeek’s model cuts costs, Nadella bets that AI’s proliferation (via tools like Copilot) will expand cloud demand, justifying Azure’s $80 billion infrastructure spend. But this assumes users won’t flock to cheaper, open-source alternatives—a risky wager.
IBM’s Hybrid Cloud Playbook
Microsoft could emulate IBM’s pivot from hardware to hybrid cloud services, blending proprietary and open-source solutions. For instance, offering premium security or integration services for DeepSeek-derived models—a strategy Red Hat used to monetize Linux.
V. Investor Psychology: From FOMO to Strategic Patience
The GameStop Effect in AI
The Nasdaq’s plunge mirrors the 2021 meme-stock frenzy, where hype detached from fundamentals. Nvidia’s 12% drop reflects panic over reduced chip demand, but history shows markets overcorrect. Warren Buffett’s dot-com era patience—avoiding overvalued tech for cash-flow giants like Coca-Cola—is a template for investors navigating AI’s hype cycle.
Amazon’s Long-Game Wisdom
Amazon survived the 2000 crash by focusing on infrastructure (AWS), not short-term wins. Similarly, AI investors should back firms building adaptive ecosystems (e.g., Google’s AI-powered search integrations) over those chasing scale alone.
VI. Workforce Strategy: Building the “AI-Native” Organization
The Industrial Revolution Parallel: From Muscle to Mind
The 19th-century Industrial Revolution replaced physical labor with machines; today’s AI revolution demands a shift from repetitive tasks to cognitive agility. IBM’s “hybrid by design” workforce—blending AI engineers in Austin with freelance data scientists in Bangalore—exemplifies this transition. DeepSeek’s rise underscores a critical truth: talent is borderless, but upskilling is non-negotiable.
Google’s “AI Residency” Model
Google’s program to train non-technical employees in machine learning mirrors Siemens’ 19th-century technical schools, which turned farmers into factory engineers. Companies must similarly democratize AI literacy, equipping marketers, HR teams, and supply chain managers to harness tools like DeepSeek’s open-source models.
Example: When Adobe pivoted from boxed software to cloud-based Creative Cloud, it retrained 90% of its workforce. AI leaders must replicate this—or risk obsolescence.
VII. Policy Imperatives: Bridging the Innovation Gap
The CHIPS Act 2.0: Beyond Hardware
The U.S. CHIPS Act’s $52 billion investment in semiconductor plants is vital, but DeepSeek proves the battleground has shifted to software efficiency. A “National AI Efficiency Initiative” could mirror DARPA’s 1960s internet project, funding open-source R&D while safeguarding IP.
Ethical AI: Europe’s GDPR Playbook
The EU’s AI Act, regulating high-risk applications, offers a template for balancing innovation and ethics. However, overregulation risks stifling agility—a lesson from China’s rapid AI adoption despite looser privacy laws.
The Manhattan Project for Algorithmic Efficiency
Just as the 1940s Manhattan Project pooled global talent for nuclear innovation, the U.S. needs a public-private coalition to counter DeepSeek’s threat. Imagine a NASA-style moonshot to halve AI training costs by 2030.
VIII. Leadership Action Plan: Thriving in the Efficiency Era
1. Adopt a “Two-Speed” R&D Strategy
- Fast Lane: Invest in algorithmic efficiency (e.g., Meta’s LLaMA 3 optimization).
- Slow Lane: Maintain core infrastructure bets (e.g., Microsoft’s Azure AI clusters).
Example: Apple’s dual focus on refining iPhones (slow) while experimenting with Vision Pro (fast).
2. Cultivate Open-Source Ecosystems
Partner with academia and rivals, as IBM did with Linux. Offer grants for developers to build on DeepSeek-like models—turning disruptors into collaborators.
3. Geopolitical Agility: The “China+1” Rule
Diversify AI talent and data centers beyond China/U.S. hubs. Samsung’s “Vietnam+Mexico” supply chain hedge post-COVID is a model.
4. Scenario Planning for Black Swans
Shell’s 1970s oil crisis playbook: Stress-test for scenarios like:
- DeepSeek 2.0: A quantum-AI hybrid emerges.
- Regulatory Shock: Bans on open-source AI models.
IX. Conclusion: The Adaptive Leader’s Mandate
DeepSeek’s disruption isn’t a anomaly—it’s the first tremor in an AI earthquake. Leaders must:
- Pivot like Netflix abandoning DVDs for streaming.
- Collaborate like Linux’s global developer army.
- Anticipate like Buffett sidestepping dot-com hype.
The companies that thrive will be those that treat efficiency not as a threat, but as a catalyst. As Henry Ford once said, “If you always do what you’ve always done, you’ll always get what you’ve always got.” In the age of DeepSeek, “what you’ve always done” is the riskiest path of all.
Dwi Suryanto, Ph.D., is a leadership and management expert, as well as a self-taught programmer. With certifications in Generative AI, LLM Apps, and AI Agents, Dwi combines his leadership expertise with cutting-edge AI technology. He also holds certifications in ChatGPT, AI for Business, and Python Programming. Through his unique blend of skills, Dwi empowers businesses and individuals to thrive in the era of digital innovation.